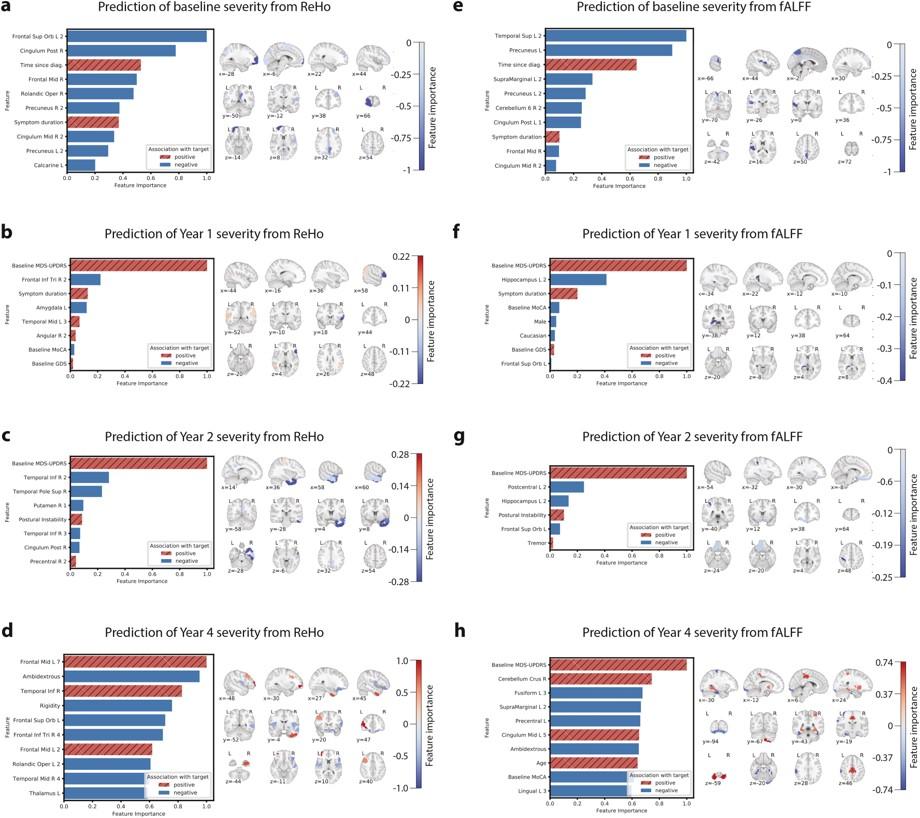
Introduction
In Parkinson's disease (PD), there is no clinically accepted neurophysiological biomarker of disease severity or future disease state [[1],[2]]. Such a biomarker would not only assist in prognosis, but also improve the design of neuroprotective treatment trials. In such trials, treatment outcomes are typically measured through clinical assessments of dopaminergic symptomatology, which do not fully quantify the underlying disease progression targeted by candidate neuroprotective drugs [[1],[2]]. A biomarker that can accurately predict future disease severity would: facilitate the enrollment of fast-progressing individuals likely to show change during the duration of a trial, empower trials to identify effective therapies, enable prognostic discussions with patients, and facilitate understanding of disease progression.
Results
Predictive performance results for each of the four MDS-UPDRS targets are summarized in Table 2. ReHo features explained 30.4%, 45.3%, 47.1%, and 25.5% of the variance in baseline, year 1, year 2, and year 4 MDS-UPDRS score, respectively. fALFF features explained 24.2%, 55.8%, 46.3%, and 15.2% of the variance in baseline, year 1, year 2, and year 4 MDS-UPDRS score, respectively. Results were significant at p = 0.001 (false discovery rate-corrected) at all timepoints except year 4, which was significant at p = 0.05. Fig. 1 plots the model prediction versus ground truth MDS-UPDRS scores for the two timepoints, years 1 and 2, at which predictive performance was highest. For classifying high-versus low-future severity, ReHo and fALFF features achieved similar accuracy, with positive predictive value (PPV) ranging from 59.4% to 78.6%.
Conclusion
Using a multivariate machine learning approach, predictors of future disease severity were developed that explained much of the variance in MDS-UPDRS total score, encompassing both motor and non-motor symptoms, in PD subjects over multiple timepoints. Measurements from a single timepoint predicted severity at current time and 1, 2, 4 years in the future. ReHo and fALFF were demonstrated to have prognostic value and important imaging and clinical features were identified, including common findings across the longitudinal timepoints, which together form a composite biomarker. The proposed set of models can be readily applied to an individual's fMRI to yield, within seconds, predictions of disease severity across several future timepoints, which helps address the need for quantitative prognostic tools. This work provides strong evidence of the value of rs-fMRI in prognosticating PD, and continued efforts to develop imaging-based tools will not only expedite treatment development, but also improve understanding of PD neurophysiology and patient care.
Source: https://www.prd-journal.com/article/S1353-8020(21)00075-4/fulltext#secsectitle0050