Analytic Methods for Determining Multimodal Biomarkers for Parkinson's Disease (U18)
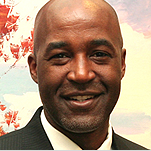
Summary
There are many exciting technological advances that provide massive amounts of medical information. Despite these breakthroughs, however, a considerable amount of this newly available information is underutilized. Better methods for using different types of information are needed to improve our ability to discover more, and to discover things faster. This is true for many scientific areas, including Parkinson's disease biomarkers research. Dr. Bowman and his team are experts in statistics, a scientific approach, in which they use mathematics as analysis tools to help them evaluate many different types of large datasets not commonly analyzed together. They are going to use this project to develop tools which will allow analysis of clinical, imaging, genetic, and biological information from subjects in the Kaiser-Permanente database and from the Emory University Morris K Udall Center of Excellence in Parkinson's disease. These tools will allow other researchers to also examine data that they collect via the NINDS Parkinson's Disease Biomarkers Program. In this way, biomarkers can be discovered that combine clinical, imaging, genetic, or other biological information, to create a constellation of biomarkers that could be useful in clinical trials and in the clinic. The methods Dr. Bowman and his team will develop will be made available to the scientific community as "freeware."